ETL (Extract, Transform and Load) pipeline process is an automated development. It takes raw data files from multiple sources, extracts information useful for analysis, transforms it into file formats that can serve business analytics or statistical research needs, and loads it into a targeted data repository.
ETL pipelines are designed to optimize and streamline data collection from more than a source and reduce the time used to analyze data. They are also designed to convert these data into useful formats before transferring them to a targeted system for maximal utilization.
Regardless of the efficiencies ETL pipelines offer, the whole purpose is lost if they cannot be built quickly and subtly. This article gives a quick guide on the necessary steps needed to build an ETL pipeline process.
Building an ETL Pipeline Process
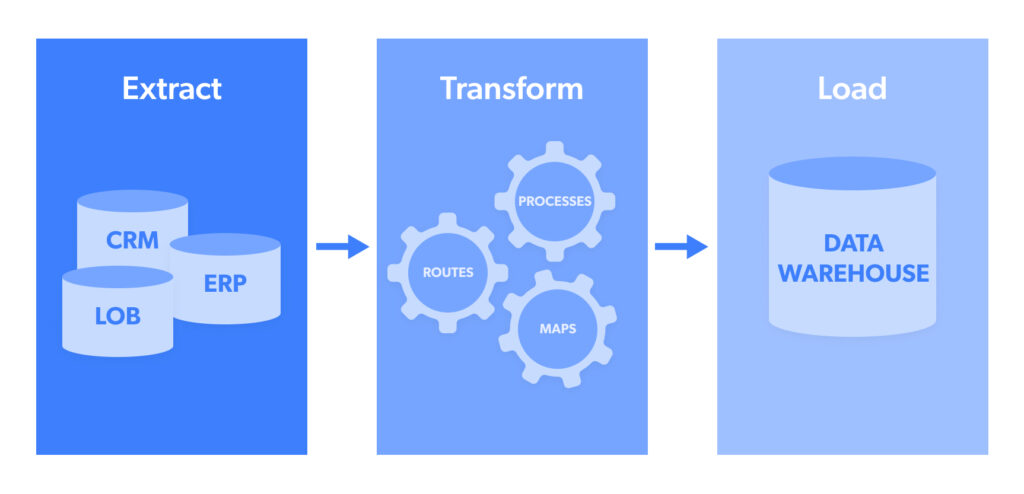
When you build an ETL pipeline, the process must be in the ETL order, i.e.
Extraction is the act of extracting data from a data pool, such as an open-source target website.
Transformation is a stage where extracted data collected in multiple formats is structured into a unique format and then transferred to a target system.
Loading is the final stage where data is uploaded to a database to be analyzed to generate an actionable output.
The ETL pipeline process is all about data extraction, copying, filtering, transforming, and loading. But when building the process, we must go through some essential details, which are as follows:
Create and Copy Reference Data
Having available raw data at your disposal can aid your vast data sourcing and solve problems in a shorter time. But if raw data is not available, you will have to create a set of data called your ‘reference data’, including a set of restricted values that your data may contain. For example, in a geographic data field, as you will specify the countries allowed, copy those data as raw records to work on while keeping the source/reference data.
Dedicate Specific Connectors and Extract Data from Available Sources
Distinct tools that establish the connection are required for data extraction from sources. Data can be stored using file formats like JSON, CSV, XML, API, etc. To systematize processing correctly, you must extract it all from a range of relational/non-relational database file format sources and convert it to a specific format to succeed in the ETL pipeline building process.
Cross-check and Filter Data
Keep relevant data and discard data that exceeds the required data range. Analyze rejected data to identify causes for rejection and make necessary adjustments to source data. For example, if you need data from the past 12 months and reject data older than 12 months to validate data, filter data by extracting off inaccurate records and null columns, etc.
Data Transformation
Data transformation is the most crucial part of the ETL pipeline process, especially when it is not automatic. The transformation process entails translating data into the desired form according to individual data use. Data must be cleansed of duplicates, checked for integrity of data standards, aggregated data, and made ready for use. Also, functions can be programmed to facilitate automation during data transformation.
Stage and Store the Transformed Data
Though It is not recommended to load transformed data into target systems directly for storage or use, instead, take the necessary step of data staging. The staging level is where data diagnostics reports are generated, and data is enabled for easy data recall in case of any havoc or malfunction. When that data is ready, it can be transferred to a target database.
Sequential Data Scheduling
Scheduling is the final stage of the ETL pipeline building process. For effective automation, it is crucial to schedule the data sequentially to load monthly, weekly, daily, or any custom time range. The data loading schedules can include a sequential time stamp to determine the load date quickly for record purposes. For efficient task automation, scheduling should be done to avoid performance and memory issues.
Conclusion
In building an effective ETL pipeline process, there is more than one method (batch processing and real-time stream processing) and data integration software that can be used. Whichever method you choose, building the ETL pipeline process in the above-listed order is the way to guarantee a successful operation.
The post Quick Guide to Building an ETL Pipeline Process appeared first on The Crazy Programmer.
from The Crazy Programmer https://ift.tt/myVFaSg
Comments
Post a Comment